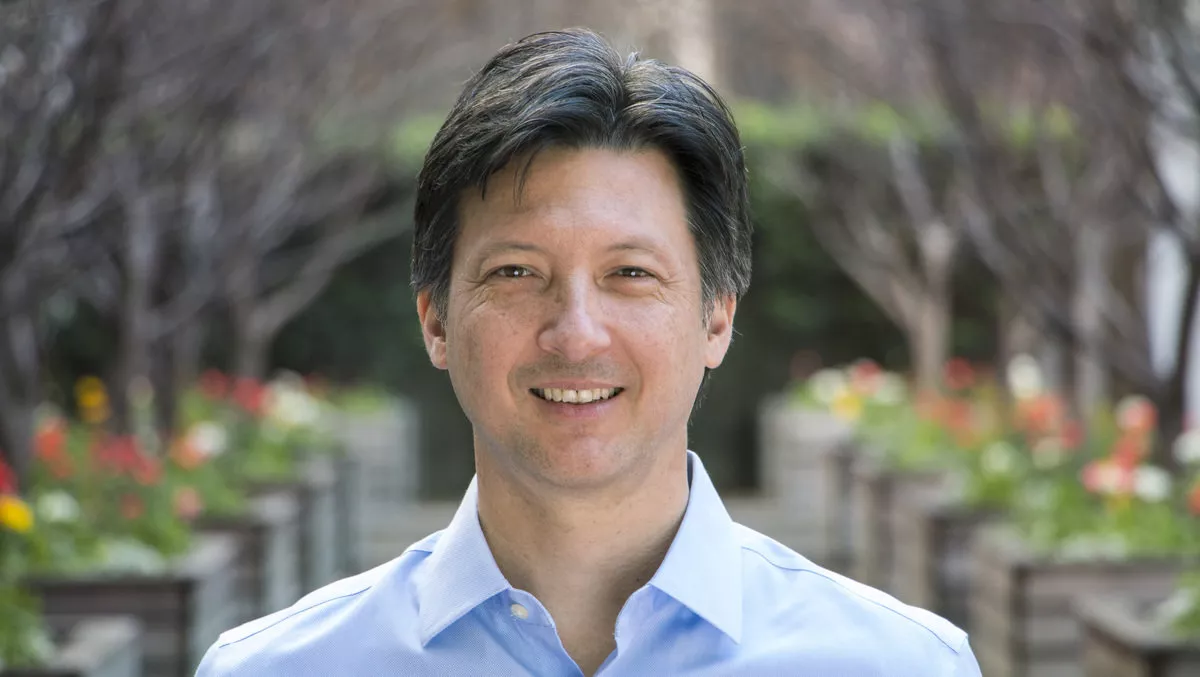
The usual AI rules still apply with AIOps - ThousandEyes
Many IT domains have been on a trajectory of increased automation and robotisation for some time.
Automation is now present in infrastructure management, in getting code to production faster, and in parts of many processes as organisations feel pressured to reduce points of friction that might cause customers to look elsewhere.
IT operations have undoubtedly benefited from this trend.
One of the reasons IT ops was ripe for automation is that environments have grown increasingly complex.
A recent survey by Telsyte found almost half (49%) of organisations in Australia use more than four cloud platforms.
The average number of cloud platforms used by organisations reached 3.8 in 2018, the survey said.
Then, there's the added complexity of all the applications that run on that cloud infrastructure, or in existing on-premises environments.
It is not uncommon to hear of enterprise environments in Australia where the total number of applications is in the hundreds or even the low thousands.
All of this adds up to a lot of alerts for IT ops to digest, as well as ongoing challenges in working out the root cause of a problem being felt downstream by end-users, whether they be internal or external customer-facing.
It's also meant that many enterprises are dealing with tool bloat.
Everyone in IT operations knows about tool bloat.
Countless studies have been done about the effects of piling on too many monitoring tools. Essentially, more tools add up to less effectiveness and poorer outcomes.
Why is tool bloat so common?
Well, let's be fair to all the engineers who have bought these tools.
There is usually a reasonably distinct data set that each chosen tool can uniquely get at, which theoretically should enhance rather than reduce operational visibility and action.
The problem is the lack of ability to correlate the signals that all these data streams provide and turn them into clear problem diagnoses and follow-on actions.
Alert fatigue is a known problem in many IT domains.
In security, for instance, a 2018 survey found 27% of IT professionals received more than one million threats daily, and 55% more than 10,000.
It's a similar story in IT ops.
AIOps Exchange recently found 40% of IT organisations receive more than 1 million event alerts each day, and 11% see "more than 10 million".
Responses to those volumes varied from tuning systems to produce less alerts, to simply ignoring whole categories of warnings.
However, some argue that alert fatigue is a strong candidate for artificially intelligent systems.
AIOps emerges - but is it the answer?
Artificial intelligence is being injected into an increasing number of IT and business domains, ostensibly as a way to take some of the day-to-day heavy lifting out of processes and ease pressure on the humans involved.
IT operations aren't immune from that trend, as evidenced by the rise of AIOps.
AIOps is a term coined by Gartner, which defines it as the "use of big data, modern machine learning and other advanced analytics technologies to directly, and indirectly, enhance IT operations (monitoring, automation and service desk) functions with proactive, personal and dynamic insight.
First, a disclaimer: ThousandEyes is not an AIOps vendor, and we're not interested in AI-washing our solution.
Our interest is in helping customers realise value from visibility.
AIOps is often pitched as a response to the fatigue-inducing correlation problem faced by IT ops staff. It uses advanced analytics to consume various streams of monitoring data and perform correlations that humans can't do via swivel-chair analysis.
AIOps is powerful and does bring real promise, but that power and promise don't come for free.
Machine learning requires learning, so who is the trainer?
The answer is that you or someone on your team will need to be, and it may take many months to teach ML something truly beneficial.
In addition, AIOps itself can't make up for significant gaps in visibility data.
The vast majority of IT ops visibility is based on passive data collection from the pieces of the puzzle it still has control over.
That's helpful data for sure.
But what about all myriads of external apps, services, infrastructure and Internet networks that IT has no direct control over and thus can't collect data on using methods?
No level of analytical intelligence can bridge this gap.
What's clear is that in order for AIOps to be effective, it needs to be fed with good data.
The AIOps platform itself is not going to be the primary monitoring data generation or collection engine.
The challenge is to get the right streams of data and to define how you want the AIOps engine to answer your questions (learning).
To get effective use from AIOps requires either thoughtful internal solution architecture work or a third party to provide pre-curated apps running on top of the platform.
SaaS like ThousandEyes are applications that are purpose-built to solve particular problems with datasets that are explicitly curated for those problems.