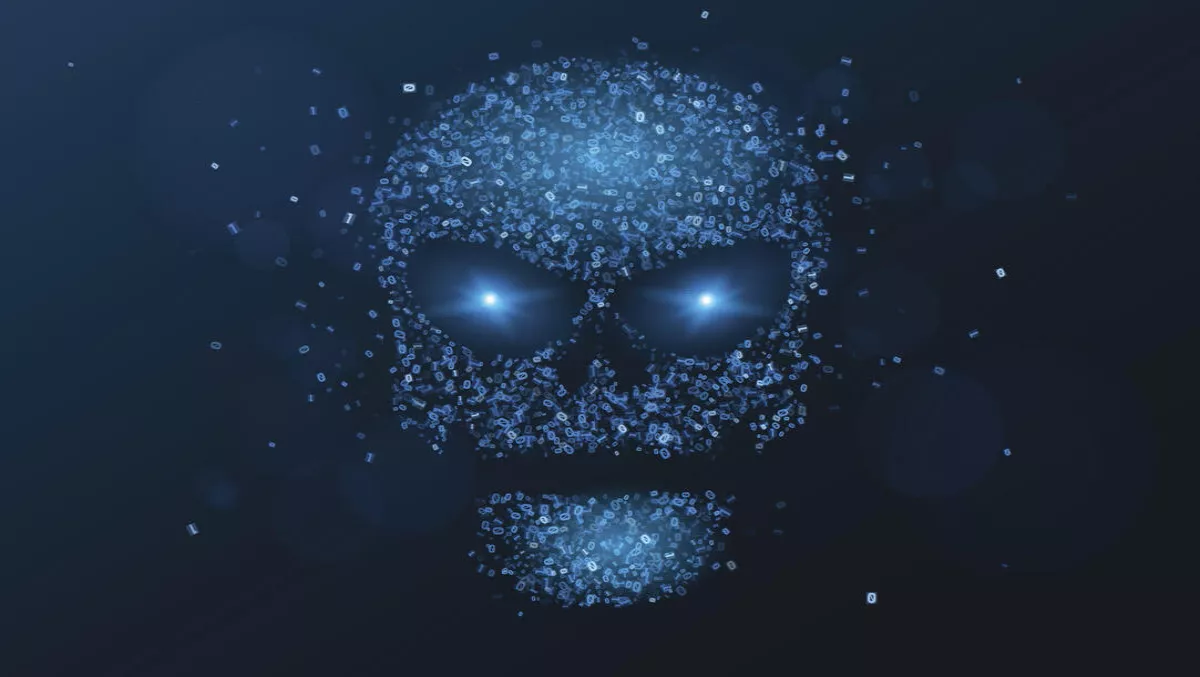
Palo Alto Networks on the three types of malware analysis & why they can work together
As IT teams and security firms formulate new ways to identify malware, criminals are also looking for ways to circumvent those processes – the time between deploying protection and criminals finding a workaround is growing shorter, according to Palo Alto Networks.
The company's ANZ regional vice president, Ian Raper, believes that there are a number of malware analysis methods, each of which has its own strengths and weaknesses.
"While the use of just one malware analysis method could leave a network exposed, implementing multiple analysis methodologies, in the right order, can give security teams a higher probability of preventing malware from penetrating the network. This includes malware samples that have not yet been identified," he says. "When implemented in series, malware analysis lets security teams handle most threats automatically, freeing up team resources to actively hunt more advanced threats.
The company states that there are three types of malware analysis to look out for: Static analysis, machine learning analysis and dynamic analysis.
1. Static analysis The first line of defence in a malware analysis environment, static analysis involves breaking down an unknown file into its component parts for examination without detonating the file.
Through static analysis, the system can determine if the file has any potential markers or patterns that would indicate it is malware. This may include embedded executable scripts or calls to connect to an unknown or suspect server.
"Static analysis is an incredibly quick and accurate way to detect known malware and variants, which make up most of the attacks usually launched against organisations," Raper says. 2. Machine learning analysis Machine learning involves creating and automating a system to classify malicious behaviour into groups. These groups can be used to identify future malicious content without needing to manually build pattern matches.
If the similarities between suspicious content are significant, the system can automatically create a malware signature and push it to enforcement points throughout the network. As further malware samples are examined and catalogued, the system's ability to mediate attacks on its own grows over time. "In today's world of commoditised cyberattacks, where even unskilled adversaries can conduct attack campaigns, machine learning-enabled analysis is one of the best methods security teams have to handle the thousands of threat alerts networks receive daily," Raper says. 3. Dynamic analysis If a suspect file cannot be handled through static analysis, it must be examined in greater detail. Dynamic analysis involves forwarding a suspicious sample to a virtual machine (VM)-based environment and then activating the sample in a highly-controlled environment (otherwise known as sandboxing), so its behaviour can be observed and intelligence extracted.
For advanced VM-aware malware, which can identify when it's being deployed in a virtual environment, bare metal analysis may be required. Dynamic analysis is particularly good at finding zero-day exploits in malware.
"Since static and machine learning analysis both require some degree of prior familiarity with the malware being analysed, it is difficult for them to identify truly novel malicious activity. The challenge with dynamic analysis is scalability, as it requires massive compute, storage, and automation resources to do it correctly," Raper says. "That said, if both static and machine learning analysis have already occurred, it is likely those processes have identified and mediated the bulk of malware to be found. Employing dynamic analysis only when needed, as part of a cloud-based automation system, effectively removes the burdens of scale and manual effort required."