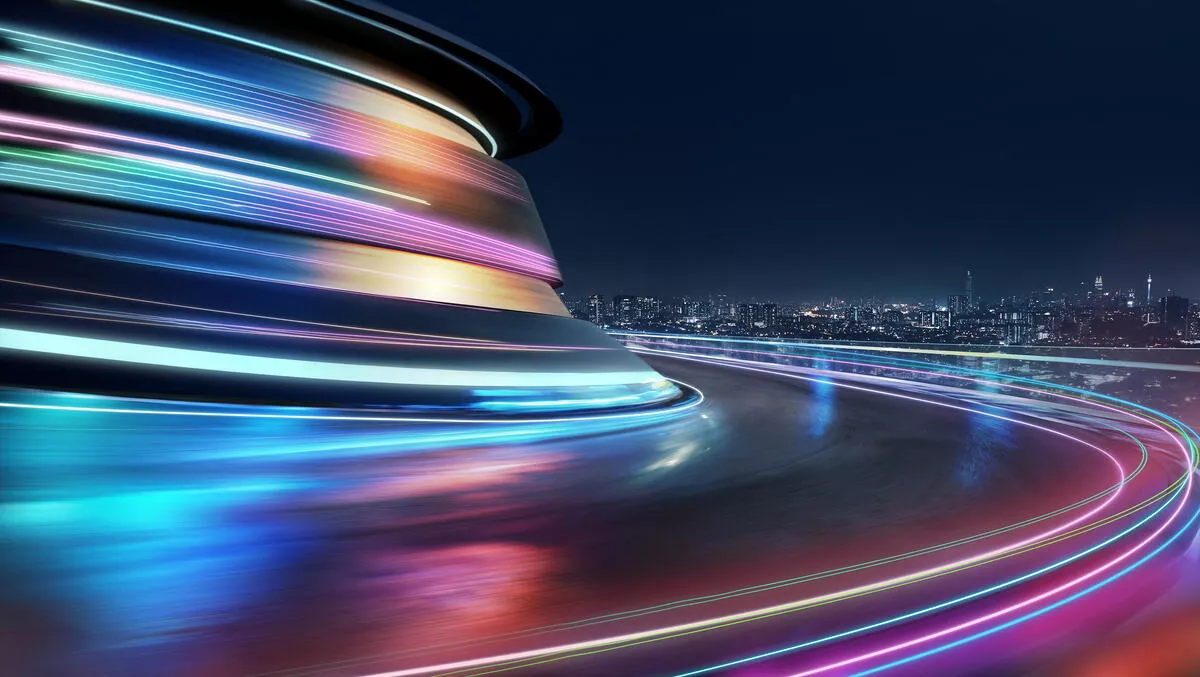
How machine learning is disrupting NGFWs and network security for the better
Machine learning has been a game-changer for almost every aspect of technology, including cybersecurity.
From cloud and software-based threat prevention to firmware in millions of security appliances, machine learning (ML) powers cybersecurity in millions of organisations around the world. Further, organisations that use ML to support their security posture have an extra edge over those that don't.
Take the traditional firewall, for example. They use rigid, set lists of rules to keep bad traffic and requests from making their way into a company's network.
But there's a problem: The IT environment - within an organisation and within the threat actor's toolbox - changes far too rapidly for a traditional security solution - firewall or otherwise - to keep up with.
Security administrators cannot keep pace with such a rapidly changing threat landscape, let alone how many devices (and what those devices are doing) on their networks. This is where ML really makes a difference. Palo Alto Networks believes it's time for security administrators to fight attackers - and the automated tools attackers use - with ML.
.Palo Alto Networks incorporates ML into its security solutions, including next-generation firewalls (NGFWs) to create a truly proactive solution for network security.
According to Palo Alto Networks, there are four key elements of an ML-powered next-generation firewall (NGFW):
1. Inline ML-powered prevention on the NGFW
Attackers will often use existing attack methods and modify them so they can slip past traditional signature-based security systems.
NGFWs can often use heuristics to detect modified malware, there always has to be a Victim 0 - a first organisation or person who experiences the attack.
Signature changes and modifications within security systems don't solve the issue, nor do alternative approaches such as analysing every bit of traffic or every file to make sure it is safe. These methods are slow and cumbersome.
NGFWs that incorporate ML can embed ML algorithms directly into the firewall's core and enforce the results, all in real-time. NGFWs can inspect a file while it's being downloaded and block anything that looks malicious before the download finishes. This is known as a single pass inspection with inline prevention, ensuring that the NGFW prevents infections without requiring any cloud or offline analysis, avoiding false positives, and reducing the potential infection to almost zero.
Furthermore, NGFWs that leverage inline ML-based prevention can also prevent threats such as fileless attacks, malicious scripts, phishing attempts, and portable executables.
2. Zero-delay signatures leveraging massive cloud scale
While ML-powered NGFWs can provide instant protection against evolving threats, sophisticated and complex threats require detection mechanisms that rely on accurate and timely signatures.
ML-based models don't cover every file format so there is a need for cloud-based analysis to support threat detection.
To solve this challenge, Palo Alto Networks stresses that ML-powered NGFWs must be paired with real-time intelligence from cloud services.
"An ML-powered NGFW reimagines and rearchitects the way signatures are delivered, once the analysis is complete and models have been updated. Instead of having to wait for a minimum of five minutes for a scheduled push, signature updates are now delivered and streamed to the connected ML-Powered NGFW within seconds, as soon as the inline ML-basis analysis is complete."
Zero-delay signatures also enable every NGFW connected to the internet to update within seconds of an initial analysis, meaning only one user sees the threat and all others are protected.
3. ML-powered visibility across IoT and other connected devices
Internet of Things (IoT) and Operational Things (OT) devices are everywhere across the enterprise. They generate vast amounts of data, which means they require protection like any other IT infrastructure.
This can be difficult given that many devices run unpatched, open source software with few (or no) security controls. As IoT devices are added to a network, the attack surface grows.
An ML-powered NGFW can classify all IoT and OT devices in a network, including new and existing devices. It is also able to leverage other IoT security technologies to provide devices based on information such as type, vendor, model, firmware, and other information.
ML-powered NGFWs can then use cloud scale to protect and manage devices.
4. Automated, intelligent policy recommendations
Networks are changing faster than security teams can keep up with, particularly if teams rely on manual tools. That can often lead to a relaxed approach with too many permissive policies.
ML-powered NGFWs can analyse huge amounts of telemetry data and recommend security policies based on their analysis of an organisation's entire network. Further, they can also use device profiles to understand normal patterns of behaviour to suggest recommended policies based on application usage, connections, and port/protocol data.
This enables security teams to take a smarter approach to policy management and saves thousands of hours of work
Why ML-powered NGFWs matter
Security threats change and evolve rapidly - for many attackers, threat creation and execution is a full-time job.
Palo Alto Networks believes that it is time to disrupt the status quo in network security by using ML-powered NGFWs to change the game.
The company's ML-powered NGFWs prevent up to 95% of zero-day file and JavaScript threats online and also use ML to create a signature delivery in less than 10 seconds.
Find out more about why ML-powered NGFWs matter: Check out the four key elements of an ML-Powered NGFW here.